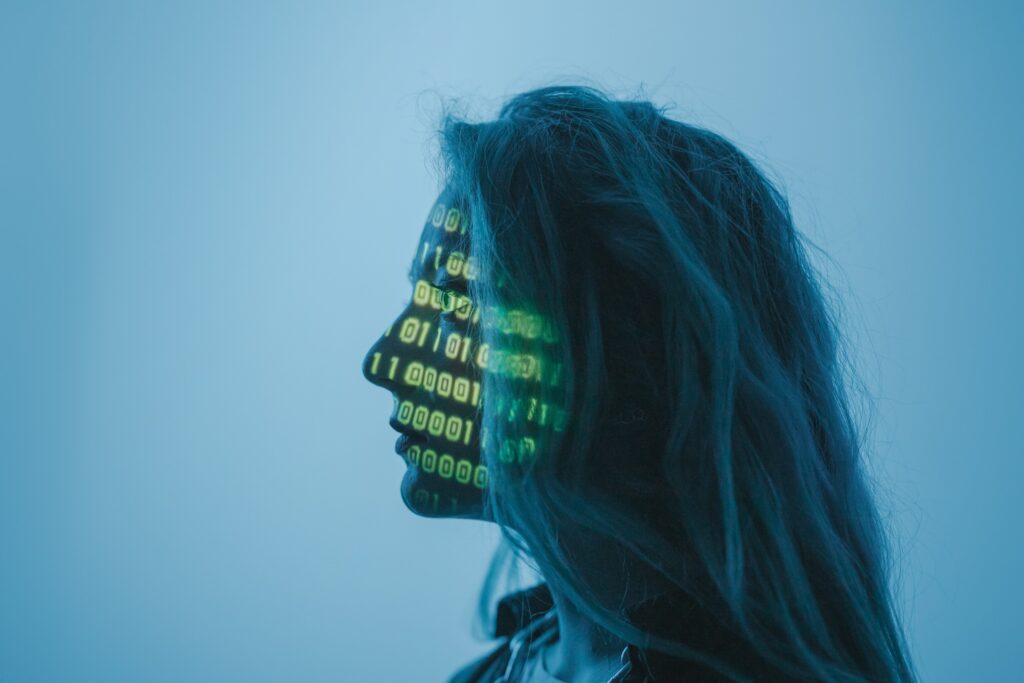
As AI becomes a part of everyday work and play, worries have also been mounting about how it influences human decisions and, on occasion, even make decisions on behalf of humans.
Much of the issue is centred on how AI arrives at a decision or recommendation, whether to tell a Tesla car to turn left or right, or to grant a bank loan or social security benefits to an individual.
Unfortunately, much of today’s AI doesn’t explain why it does what it does. Such “black box” AI is either too complex or it may have come from proprietary sources – whether through its training data, model or algorithm – so tracing back a recommendation or decision is near-impossible.
Yet, many in the AI field, particularly those not in the current Big Tech bubble, have been working with some form of explainable AI for a while now. With much higher stakes involved, they have been using “glass box” AI that produce results that can be more transparently explained.
One company is Beyond Limits, a company co-founded in 2014 by engineers who had worked on AI at the NASA Jet Propulsion Laboratory and the California Institute of Technology (Caltech). Its AI technology includes intellectual property licensed from NASA’s R&D investments over the years.
Today, the company uses its so-called cognitive AI technology to help oil and gas companies run their refineries optimally and safely. To produce, say, a lubricant for a new car engine, Beyond Limits’ AI also helps find the best formula to produce the best results.
“Most AI companies out there are typically pure machine learning – 95 per cent of the companies out there basically take and ingest tons and tons of data, look for patterns and then try to predict the future based on the history,” said Leonard Lee, president of Beyond Limits in Asia-Pacific.
“But it is really difficult in highly industrialised areas like oil and gas or advanced manufacturing because usually data is not complete or labelled or you have inconsistent data,” he added.
“Before the machines and all this AI stuff, it was human beings looking at the situation on the refinery floor or the manufacturing floor, and then making a decision based on years and years of experience, judgment, intuition and experience,” he noted.
“Basically, we have codified some of this human knowledge and human experience. And then we combine it with machine learning to then produce the best decision recommendations for the operator, whichever use case it is,” he added.
“That process is super complicated. The refinery size of 10 football fields, two million sensors, terabytes of data being pumped out from those sensors, and you still must figure out what is the most efficient way to run the refinery,” he explained.

Most importantly, a recommendation can be traced back to how it was derived, whether this is from historical data from sensors, safety guidelines in manuals or e-mails between supervisors and operators.
While the AI won’t directly refer to a particular e-mail, it could say a recommendation came from “cognitive traces”, in the form of different conditions, set points and policy guidelines.
“Operators, when they are given decisions or recommended decisions, can track and trace why the machine recommended a certain decision, and what the conditions were that triggered those recommendations,” said Lee.
“On the other hand, as we all know, when we use ChatGPT and most other AI tools, you don’t know why they recommend that solution or the answer to you,” he added. “It’s a black box, and you can’t trace back on those conditions.”
Besides the oil and gas industry, Beyond Limits has expanded into healthcare and financial services as well. In each of these highly regulated industries, businesses are keen to embrace AI but also aware that they have to get things right.
Explainable AI could help a radiologist explain how an AI helped him come to a conclusion that a tumour was present, for example. At a bank, the lender can be more transparent in explaining why it had offered or declined credit facilities to a company.
“If you want to trust a prediction, you need to understand how all the computations work,” said Professor Cynthia Rudin at Duke University, who specialises in interpretable machine learning.
“For example, in health care, you need to know if the model even applies to your patient,” she told Quanta Magazine in an interview in April this year. “And it’s really hard to troubleshoot models if you don’t know what’s in them.”
However, making explainable AI the norm, even as Big Tech firms zoom ahead with their ever more powerful tools today, is not going to be easy, say AI researchers.
One obstacle is the lack of consensus of even the definition of key terms. Precise definitions of explainable AI vary, according to Violet Turri, an assistant software developer at the Carnegie Mellon University.
“Some researchers use the terms explainability and interpretability interchangeably to refer to the concept of making models and their outputs understandable,” she wrote last year on the university’s blog. “Others draw a variety of distinctions between the terms.”
Tough as it may seem, making sense of what an AI does under the hood is going to become more important in the years ahead. Its decisions are going to be under more scrutiny, as they impact people in more profound ways than ever.
In an ongoing trial in the United States, victims of a fatal crash of a Tesla car have blamed the car marker for its Autopilot driver assistant system.
In the crash in 2019, Micah Lee was killed and his two passengers, including an eight-year-old boy, were seriously injured. The car had veered off a highway east of Los Angeles, struck a tree and burst into flames in mere seconds, reported Reuters.
Tesla, for its part, has blamed human error for the crash, questioning if the autopilot was engaged at the time of the crash. Tellingly, despite its name, the autopilot feature still needs a human to be alert at the wheel.
The trial, which began two weeks ago, is expected to take several weeks and could impact self-driving car technology in the coming years as well as trust in AI, in general.