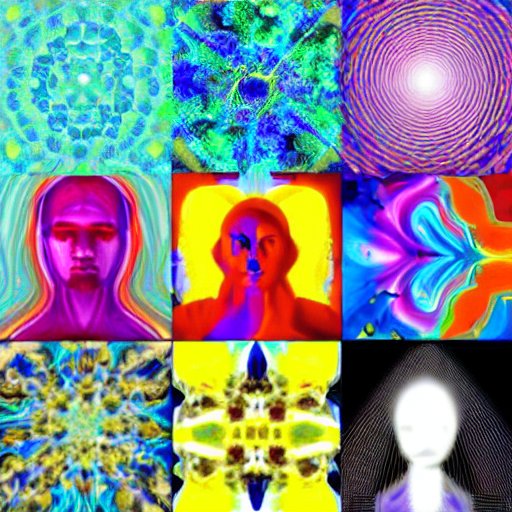
By Professor Virginia Cha
It is difficult for us to grasp the exponential speed at which AI tools are developing more advanced and powerful capabilities. It only took five days for ChatGPT to reach one million users, two months to reach 100 million MAU (Monthly Active Users). This is faster than any other technology adoption known to mankind.
When a change happens at such an exponential speed, something will break. Just look at the recent demise of Silicon Valley Bank (SVB). When the US Federal Reserve raised interest rates from 0.25 per cent to five per cent between March 2020 to March 2023, it created enormous stress in the banking sector and caused the second largest banking failure in the U.S.
We may think of it as an increase of 4.75 per cent in interest rate, but this is a 4900 percent, or 49 times increase over two years. It’s not the nominal change that matters, it’s the proportional exponential change that breaks things. We’ve built systems that assumed a more gradual change over time.
Hence, we must be ready for the breakage that will arise from these new Large Language Model (LLM) tools such as ChatGPT. There are many tools available – here’s a list of tools you can use to acquire super-human skills.
Most of the key skills converge into one issue: the ability to manipulate and generate language, whether in words, sound, images or moving pictures. Why is this such a fundamental change and what does it mean for people?
Language is the core centre of human culture. Language, for example, allows us to articulate laws, money systems, art, science, sovereignty and computer code. AI’s new mastery of language means it can manipulate the core of our social, economic, political and business operating systems.
What would it mean for us where a large percentage of our news, images, music, stories, laws, policies and software are created by Generative AI?
One can argue that the works from these tools can be generated with supersonic efficiency and without the weaknesses and biases of the human mind. But humans have always excelled in the arts and culture, and all things that require emotions. Now even emotions can be mimicked by generative AI.
An Alibaba research unit recently developed an AI model called model scope that creates short two-second videos based on written text prompts. The Alibaba affiliated company Huggingface has made this tool available.
This interesting tool has two immediate implications. First, the race to dominate AI technology is global. Second, what human invented organisations would not be necessary now?
For example, do we still need to hire an advertising agency to run our next marketing campaign, with all the associated costs of a video production team, performing actors/actresses and script writers when we can just generate the next more effective advertisement in the space of a few minutes and less money than the price of coffee?
Perhaps in the next US Presidential election, entire campaigns can be run by an AI. Can AI run the Singapore government or any government? Perhaps super smart civil servants are not needed. Instead, very smart Prompt Engineers (and I don’t mean engineers who are on-time) are required.
Things will break. As a society, we need to develop new tools to anticipate the breakages, so we can all benefit from the exponential rise of large language models and AI.
The reason why there are breakages such as the SVB failure is because the current systems have been designed using the Plan-Do-Check-Act (PDCA) method for a more stable PESTLE (Figure 1) world where technology adoption rate is at a pace relative to how social, economic and political systems can absorb the changes introduced by the innovation.
The PDCA cycle has been in coexistence with the more recent highly optimised Lean/Agile method, but at its fundamental level, it is still PDCA on cheap steroids. We need a different mindset -one that embraces and anticipates systems breaking and be able to benefit from a new and emerging system.
A new way of thinking which embraces the idea of Agility, Ambidexterity, and Antifragility (Figure 1), which enables smart decisions in the face of deep technology changes. This will enable people and society to become ever stronger and allow future new systems to emerge.
We need to have multiple methods for assessing and realising the opportunities from deep technology changes, and depending on the environmental (PESTLE – see Figure 1) context, we switch to the best tool. For example, if you are a researcher working on therapeutics for immunology, and you are assessing how generative AI will impact your work, then you can consider the Agile method, as the environment for drug development, testing, and regulatory approvals are stable.
If you are working in fintech, then you should consider Antifragility, because you are working in a high-flux environment with multiple changes such as regulatory interventions and the fragile banking system are at play.
Antifragility is the ability to profit from systems breaking – and the core of it requires you to develop multiple options, or small bets on the possible outcomes, with a mindset of affordable loss if the options do not realise. However, if one of the bets comes true, then the exponentiality of the change can bring you outsized returns on your efforts.
This is a call to action to all educators, teachers, and knowledge facilitators. Teach with the future and use the appropriate LLM as the core to your curriculum. Just two decades ago, when the smartphones emerged as the global phenomenon, young people would say, “here’s an app for that”. Today, there’s an LLM appropriate to your problem domain and we will say, “there’s a LLM for that.” If you don’t know which one, just ask ChatGPT.
Like bodybuilders, we need to break down our muscles to rebuild stronger bodies. Let us embrace generative AI as a society, and to build up our people to be ready for the age of breaking things.
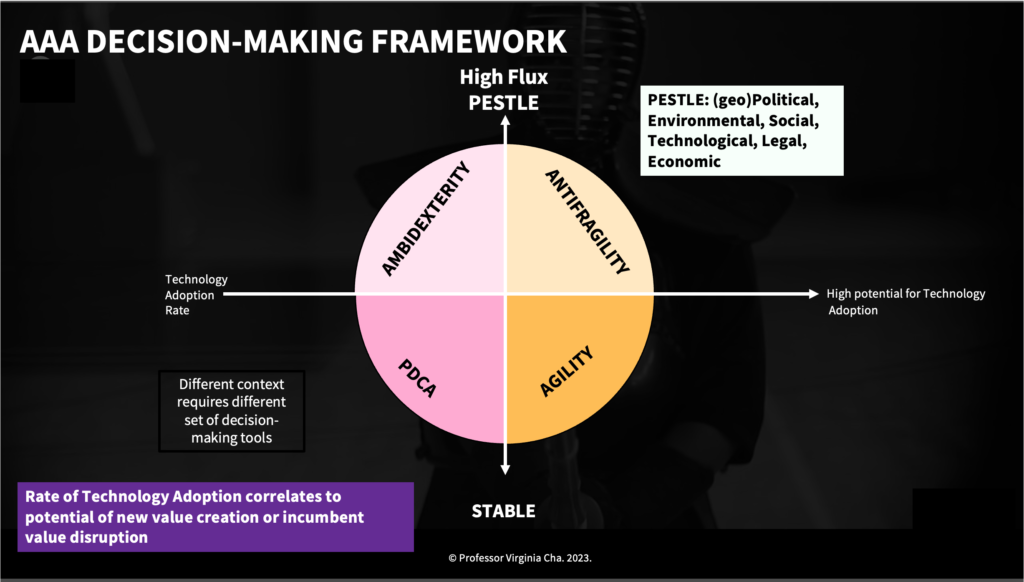
Professor Virginia Cha has a multi-faceted 40-year career in industry and academic in Singapore, China, United States and other countries. She has founded and listed tech companies in the US and Hong Kong.