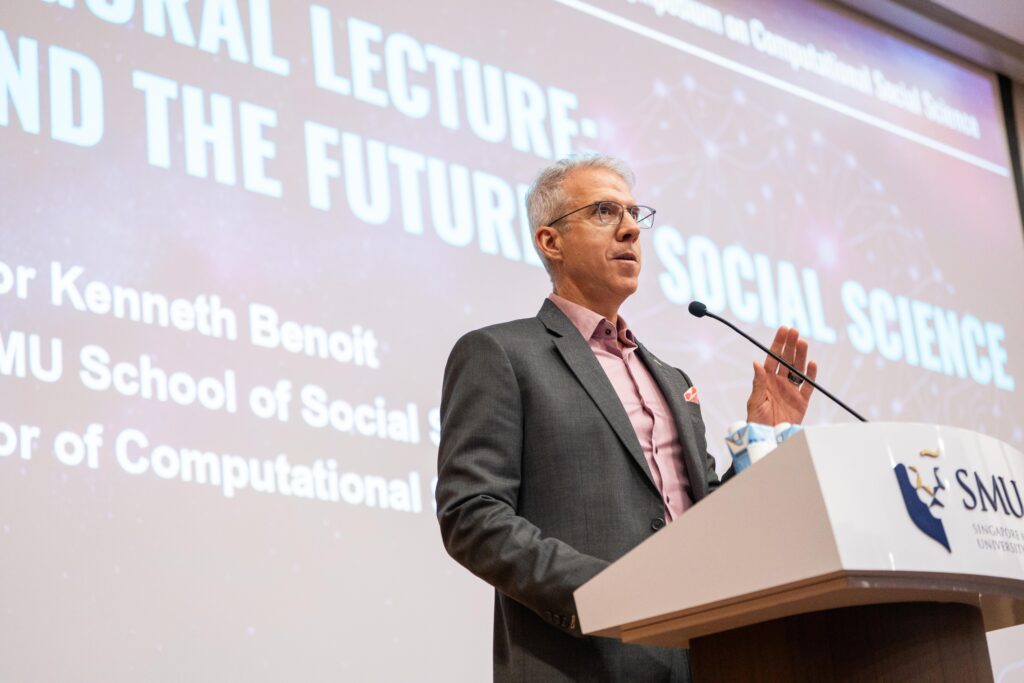
IMAGE: Deeptech Times
AI is impacting social science educators and for students of social science itself. Prof. Kenneth Benoit, Dean, SMU School of Social Sciences, identified three areas—knowledge transmission, knowledge production, and knowledge application—where AI is expected to have the largest impact. Benoit was delivering his inaugural lecture AI and the Future of Social Science as Professor of Computational Social Science at Singapore Management University (SMU).
Identifying the key disciplines that social science comprises, Benoit said: “At one end, we have economics, followed by the empirical disciplines of psychology and political science. At the other end, we have interpretative disciplines such as sociology and anthropology, with other disciplines such as geography and communications studies somewhere in between. Philosophy also occupies the interpretative end of the spectrum.”
“All of these disciplines lie, broadly, to the right of the STEM fields—where the focus is on product rather than process—and to the left of the humanities, which focus on meaning than measurement. Where a discipline sits along that spectrum helps determine how AI is reshaping it.”
In more technical fields such as economics or computational sociology, Benoit said that AI tends to act as an evolutionary force. It speeds things up, scales things out, and makes established methods more efficient without altering the underlying fundament.
Move towards the interpretative end like history and anthropology, and AI is potentially revolutionary. “Here, it doesn’t just assist with the work; it has the potential to replace the very process of scholarship,” said Benoit. “When LLMs, or large language models, generate arguments, simulate positions, or analyse culture, they don’t just support interpretation—they may begin to supplant it.”
For knowledge transmission, he sees student use of AI change how learning takes place. “With the advent of generative AI, we are seeing a subtle but profound shift. Students can now query an LLM to explain a concept, summarise a theory, or even produce a first draft of a paper. The result is a learning cycle where AI inserts itself between the student and the task of synthesis,” Benoit explains.
What was once a process of knowledge construction may become one of knowledge confirmation. The question then is not only what is learned, but who, or what, is doing the learning. In other words, is it the student learning, or is it the tool?
On knowledge production, while LLMs shorten the process of research and interrogation in learning, it potentially subsumes the user’s own expertise, reducing them to being merely machine operators. Benoit warns that as “AI becomes more fluent in these tasks, we risk losing the nuance and depth that come from human engagement.”
He argues that as AI becomes more powerful, we may find it increasingly replacing the human judgment, interpretative labour, and creative analysis that lie at the heart of qualitative disciplines. This threatens the value of those fields not because their subjects are disappearing, but because their methods—the human processes of inquiry—are being displaced.
In essence, we risk losing an essential human reflex to interpret and attach meaning to AI-crafted outputs.
For knowledge application, Benoit notes that AI could reshape what counts as actionable knowledge, and how much we trust that knowledge to guide decisions in the real world. “Large language models are trained on a vast archive of human expression—our speech, our writing, our decisions, our creativity,” says Benoit.
“They’re not trained on reality. They’re trained on what we’ve already said about reality. That makes them deeply derivative. And increasingly, the danger is that we don’t just train the model on life—we start, in effect, training life on the model.”
He highlighted leakage in AI as a serious issue. Leakage occurs when a model inadvertently learns from information it shouldn’t have access to—data it wouldn’t encounter in the real world. This could lead to unintended consequences where for instance, GenAI produces answers it was previously trained, but will fail to do the same in future situations that were not part of this training data.
“The problem of data leakage is more insidious than just AI cheating on performance benchmarks. It’s what happens when the exam isn’t just being answered by AI—but written by it in the first place,” said Benoit.
Looking ahead, Prof. Benoit thinks social science is critical to enhancing societal value – by asking the right questions of AI, applying critical thinking skills, and providing a human interpretation (emotional, empathetic) of AI outputs. “[I want to] highlight both the vast opportunities—and the serious challenges—that AI brings to the social sciences. If we act wisely, we can harness these extraordinary tools for a healthy and productive process of continued knowledge transmission, production, and application,” said Benoit.
“To make effective and safe use of these technical tools, we must, just as urgently, defend and renew the skills at the interpretative end—skills of judgment, critique, interpretation, and ethics. Because in the end, these are the contributions that only humans can make.”
At its core, can social science help increase trust in AI through ethical AI guardrails, while also challenging society’s unquestioning reliance on AI?