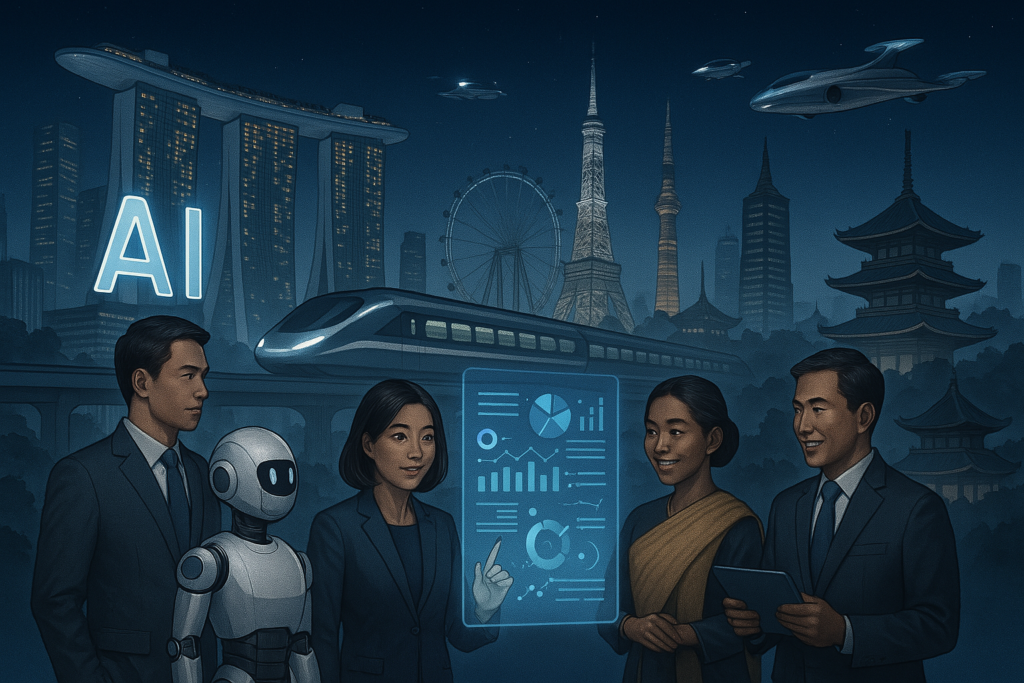
The Stanford Institute for Human-Centred Artificial Intelligence (HAI) has published its 2025 AI Index Report. Each year, the report covers significant technical advances, benchmarking achievements, investment trends in GenAI, education developments, legislation related to this technology, and more.
Here are five key takeaways:
Asia is more optimistic about AI
Regional differences remain regarding AI optimism. In countries such as China (83 per cent), Indonesia (80 per cent), and Thailand (77 per cent), a large majority believes AI-powered products and services offer more benefits than drawbacks. This view is less common in Canada (40 per cent), the United States (39 per cent), and the Netherlands (36 per cent).

Smaller models improve
In 2022, the smallest model achieving a score above 60 per cent on the Massive Multitask Language Understanding (MMLU) benchmark was PaLM, with 540 billion parameters. By 2024, Microsoft’s Phi-3-mini, with 3.8 billion parameters, reached the same threshold. This marks a 142-fold reduction over two years.

Models get cheaper to use
Querying an AI model with GPT-3.5-level accuracy (64.8 per cent on MMLU) dropped in cost from US$20 to US$0.07 per million tokens between November 2022 and October 2024—a 280-fold decrease. LLM inference prices now vary, dropping 9 to 900 times annually, depending on the task.

Business invests in AI usage to boost productivity
In 2024, U.S. private AI investment reached US$109.1 billion, significantly higher than China’s US$9.3 billion and the U.K.’s US$4.5 billion. GenAI attracted US$33.9 billion globally in private investment, marking an 18.7 per cent increase from 2023.
The use of AI in business is also increasing: 78 per cent of organisations reported using AI in 2024, compared to 55 per cent in the previous year. A growing body of research indicates that AI enhances productivity and helps address skill gaps across the workforce.
Complex reasoning remains a challenge
AI models excel at tasks like International Mathematical Olympiad problems but struggle with complex reasoning benchmarks like PlanBench. They often fail to solve logic tasks consistently, even when solutions are known, limiting their effectiveness in critical settings requiring precision.

Read the full report here.